By David Calvert, October 2024
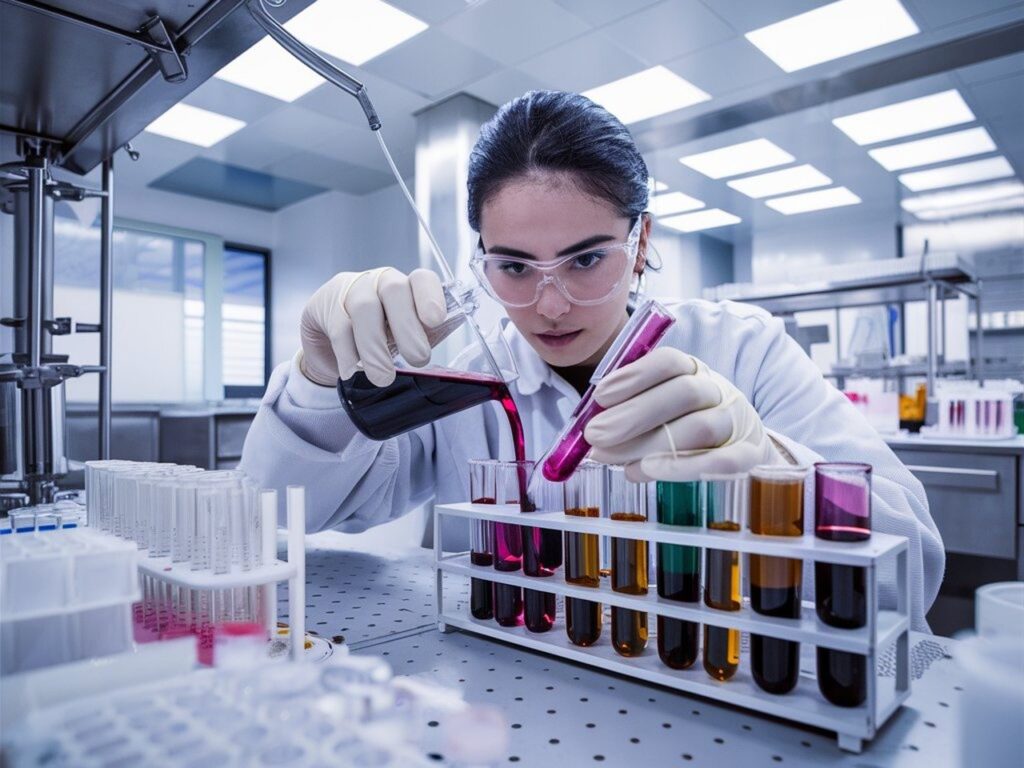
It has been a while since the last post on our approach to Design for Formulation but now we come back to an element that is common to all science projects, namely experimental design.
So, there can be many ways of approaching your design and in many cases you use a combination of these approaches (after all a formulator is not a robot, powered by AI!). We often talk about five approaches but in recent times a sixth has been developed, but more about that later. The first approach (we call it Trial and Error) involves a large amount of risk but is based often on experience in that a formulation worked well in the past and we start from there. This “bottle picking” can be successful but any advances are likely to just be incremental and not revolutionary.
The second approach moves up the scale and again relies upon experience but combines this with some rules of thumb, such as recommendations from a supplier, or an understanding of ingredients having worked previously with a chemically similar active ingredient. The next level of complexity involves Statistical Design of Experiments and this approach has been made much more accessible with software developments. Caution is still required and you should avoid using this as a “black box” and not giving findings some scrutiny. We have worked with clients where the noise from the test method has exceeded the relationship they were claiming. A good statistical design and analysis can help you to reach the global optimum formulation, however, and bring some radical understanding and changes. You can listen to an old webinar with more details on Statistical Design of Experiments on our web-site.
A modification of the rule of thumb approach is to make use of some theories and models that have tried to rationalise results. This could be the use of Hydrophilic Lipophilic Balance (HLB) to help with choice of surfactant or Stokes Law to improve stability of suspensions and emulsions. Another theory that we have found useful has been Hansen Solubility Parameters (HSP). We have a webinar recording on our web-site which explains more on HSP.
The most complex approach to implement is the use of fully predictive models for formulation. This “in silico” formulation has been investigated most in pharmaceuticals and can be costly although it can lead to the evaluation of more radical formulations.
It is impossible to discuss any topic these days without mentioning the use of artificial intelligence and as we have discussed a number of times, this is being looked at by formulators. An interesting approach using machine learning is being developed by Intellegens and their system can use sparse, noisy data to develop further experiments and optimise formulations. All of course still need the principles we have outlined in previous posts on Design for Formulation such as customer promise, critical attributes and risk assessment.